D-Lib Magazine
Measuring Scientific Impact Beyond Citation Counts
Robert M. Patton, Christopher G. Stahl and Jack C. Wells
Oak Ridge National Laboratory
{pattonrm, stahlcg, wellsjc}@ornl.gov
DOI: 10.1045/september2016-patton
Oak Ridge National Laboratory
{pattonrm, stahlcg, wellsjc}@ornl.gov
DOI: 10.1045/september2016-patton
Abstract
The measurement of scientific progress remains a significant challenge
exasperated by the use of multiple different types of metrics that are
often incorrectly used, overused, or even explicitly abused. Several
metrics such as h-index or journal impact factor (JIF) are often used as
a means to assess whether an author, article, or journal creates an
"impact" on science. Unfortunately, external forces can be used to
manipulate these metrics thereby diluting the value of their intended,
original purpose. This work highlights these issues and the need to more
clearly define "impact" as well as emphasize the need for better
metrics that leverage full content analysis of publications.
exasperated by the use of multiple different types of metrics that are
often incorrectly used, overused, or even explicitly abused. Several
metrics such as h-index or journal impact factor (JIF) are often used as
a means to assess whether an author, article, or journal creates an
"impact" on science. Unfortunately, external forces can be used to
manipulate these metrics thereby diluting the value of their intended,
original purpose. This work highlights these issues and the need to more
clearly define "impact" as well as emphasize the need for better
metrics that leverage full content analysis of publications.
1 Introduction
Measuring scientific progress remains elusive. There is an intuitiveunderstanding that, in general, science is progressing forward. New
ideas and theories are formed, older ideas and theories are confirmed,
rejected, or modified. Progress is made. But, questions such as how is
it made, by whom, how broadly, or how quickly present significant
challenges. Historically, scientific publications reference other
publications if the former publication in some way shaped the work that
was performed. In other words, one publication "impacted" a latter one.
The implication of this impact revolves around the intellectual content
of the idea, theory, or conclusion that was formed. Several metrics such
as h-index or journal impact factor (JIF) are often used as a means to
assess whether an author, article, or journal creates an "impact" on
science. The implied statement behind high values for such metrics is
that the work must somehow be valuable to the community, which in turn
implies that the author, article, or journal somehow has influenced the
direction, development, or progress of what others in that field do.
Unfortunately, the drive for increased publication revenue, research
funding, or global recognition has lead to a variety of external factors
completely unrelated to the quality of the work that can be used to
manipulate key metric values. In addition, advancements in computing and
data sciences field have further altered the meaning of impact on
science.
The remainder of this paper will highlight recent advancements in
both cultural and technological factors that now influence scientific
impact as well as suggest new factors to be leveraged through full
content analysis of publications.
2 Impact Versus Visibility
Prior to the development of the computer, Internet, social media,blogs, etc., the scientific communities' ability to build upon the work
of others relied solely on the specific and limited publication venues
(e.g., conferences and journals) to publish and subscribe. With limited
venues, the "noise" of excessive publishing was restricted and
visibility of scientists across their respective fields was not
diminished by this. Consequently, the clearer the visibility of work
that is published by the respective scientific communities, the cleaner
the interpretation of citation analysis and the stronger the association
between citation and "impact".
Unfortunately, the "noise" of publications has increased
significantly such that some fields are doubling their total publication
count every fifteen years or less.[10]
Over the course of the last 100 years, much of this growth rate could
be attributed to a variety of factors such as the advent of computers,
Internet, social media, blogs, to name a few. In fact, a new field,
altmetrics, emerged in 2010 [14]
for the sole purpose of attempting to measure "impact" of a publication
via these additional factors. Further, another factor in influencing
the growth rate of publications is an increasing level of focus on
requiring justification for the investments made in scientific
research.[2] In support of this increasing focus, a new set of metrics are being formed, snowball metrics [3], that seek to assess research performance using a range of data not just citation data.
While these two new areas, altmetrics and snowball metrics, are
attempting to gain clarity on assessing impact as a result of the
increasing "noise" of publishing, they are, unfortunately, not truly
addressing the noise problem, nor do they reach into the heart of the
matter, namely, does a researcher's content alter the progress of the
scientific community? Ultimately, the vast majority of these metrics are
simply indirect means of evaluating content. The underlying
assumption remains: if a scientific publication references former
publications, then a human researcher has determined that the former publication in some way shaped the work that was performed. Therein lies the problem. Indirect
measures, along with increased pressures for assessing performance,
means there are simply too many different ways of manipulating these
metrics and their corresponding conclusions. In fact, there are
publications that discuss such approaches. [4]
identifies 33 different strategies that researchers can utilize to
increase their citation frequency and thus their impact measure. These
strategies involve increasing a researcher's visibility, and not
necessarily the quality of their work. As stated by the authors, "The
researchers cannot increase the quality of their published papers;
therefore, they can apply some of these 33 key points to increase the
visibility of their published papers."
There is some evidence to suggest that increasing visibility does, in
fact, increase impact more broadly. In the work performed by [20],
the authors performed a full content analysis of citing/cited article
pairs. They found that there is "a general increase in explorative type
citations, suggesting that researchers now reach further into our
collective "knowledge space" in search of inspiration than they once
did." In addition, they found that over time, the distance between the
full content of these pairs grew, suggesting more interdisciplinary work
was being performed. There are two key aspects about the work performed
by [20] that are worth noting. First, it was only through a full content analysis
of the publications that these conclusions could be drawn that
citations are reaching more broad areas of research. Second, it does
suggest a positive correlation between visibility and impact. Higher
visibility leads to higher impact via increased multidisciplinary
citations. However, despite these two key aspects, we are still left
with several challenging questions: did a publication truly have an
impact as opposed to simply being highly visible, and if so, how?
Unfortunately, efforts to effectively measure impact appear to only
expand the entanglement between research funding, impact, and
visibility.
3 Challenges of Defining & Measuring Impact
As evidenced by the formation of [2],increasing pressure to justify investments made in scientific research
require a more quantitative approach to identifying return on
investment. Unfortunately, this means determining an objective,
quantifiable measure for something that is often subjective and
qualitative. Before addressing that challenge, we must first identify
what is meant by "impact".
There have been previous works that have studied the definition of
impact and the various factors to consider. As an example, in the work
of [5],
the authors identify different types of impact beyond economic impact
as well as different indicators for each type. Despite the attention to
identifying different types of impact, many of the indicators are based
on citation analysis. Unfortunately, the flaws of citation analysis have
been identified [11][12][13],
and with no clear solution or alternative approach. The primary issue
with citation analysis is that there are simply too many assumptions
that are made and most of those are assumptions about human behavior or
human interpretation. In the work of [19],
the authors explored the quantification of impact beyond citation
analysis. However, their approach relied on a wide range of experts to
review the content for a very specific field. Unfortunately, as the
authors noted, their method "is time consuming to carry out as it
requires a thorough literature review and gathering of scores for both
research publications and interventions or solutions." Consequently,
there exists a gap in attempts to measure impact. On one hand, there is
citation analysis that can be easily tracked and automated, but relies
on many assumptions that are easily manipulated. On the other hand,
there is a more rigorous full content analysis by a panel of experts,
but relies on the availability of those experts as well as an enormous
amount of time to perform.
Our premise here, very simply, is that "impact" is defined as the
level to which one resource is required by another resource in order to
produce an outcome. Seminal publications epitomize this definition in
that they are publications on which all others form their basis, and are
usually considered to have made a significant contribution to the scientific body of knowledge. Most citation analysis and altmetrics focus on quantifying the "level" aspect of our definition. In works such as [5],
others have worked to define the "outcome" aspect of our definition.
What is missing is the need to more clearly define the "resource" aspect
and the "required" aspect of our definition. How do we define these
aspects?
3.1 Resources & Requirements for Scientific Progress
While publications of prior research is a primary resource on whichnew research is performed, today's scientific communities rely on a
multitude of other resources beyond publications for example:
- High performance computing facilities such as the Oak Ridge Leadership Computing Facility (OLCF) that enable advanced simulations of a wide range of natural phenomena
- Visualization laboratories such as the one at the Texas Advanced Computing Center (TACC) that enable advanced visualization of scientific data sets
- Specialized instrumentation facilities such as the Spallation Neutron Source (SNS) that enable the interrogation of advanced materials at the atomic level
- Data centers such as the Distributed Active Archive Center (DAAC) that provide the capability to archive and management scientific data and models
- Models of natural phenomena such as the Community Earth System Model that enable the simulation and study of these phenomena
- Databases of measurements and experimental results such as MatNavi that enable scientists to search for various properties of different materials
and ultimately publish their work. In many cases regarding these types
of resources, the research performed simply would not have occurred if
the corresponding resource did not exist or was not accessible. While
these resources are not publications, they typically have corresponding
representative publications that can be cited so as to provide "credit
where credit is due". If they do not have a corresponding publication to
cite, then they will have an acknowledgment statement that they request
be used for any publication that leverages their resources.
Unfortunately, in the case of acknowledgment statements, citation
analysis simply does not provide appropriate attribution to assessing
the level of impact. For many of these resources, such acknowledgments
or citations are critical to continue receiving their operating funding levels and justify the financial investment made to create them.
When we investigate the use of these resources and the manner in
which they are cited or acknowledged, a tremendous gap exists with
respect to assessing impact. There are two issues:
- Not all cited works provide an equal contribution to a publication
- Not all required resources are provided appropriate credit or even credit at all
process that human experts can identify very easily, but requires
significant time. In the first issue, some cited works are provided
merely for reference or background purposes for the reader while other
cited works are so critical to the citing work that the citing work
would probably not have even existed if not for the existence of the
cited work. However, citation analysis treats both citations equally,
which is clearly not an accurate assessment of impact. In other words,
some cited resources are required for the citing work to exist
while others are not, but citation analysis alone does not make this
distinction. Citation analysis only considers published resources and
not other types of resources thus impact assessment is not complete. In
the second issue, there does not exist any formal, standard means of
citing such resources other than through a corresponding publication.
This is the reason why some resources have resorted to publishing a
corresponding paper so as to have some means of being cited for their
contribution and they request their users to cite their paper. Citation
analysis is then used on that corresponding paper in order to measure
"impact". Some resources have attempted to create ARK (Archival Resource
Key) Identifiers as a means of being cited [1].
However, this is not the intended purpose for ARK Identifiers and does
not enable the benefits that may be achieved through citation analysis.
4 Toward Full Content Analysis to Assess Impact
As identified by [19]and the issues stated previously regarding scientific resources,
additional information and value from publications, with respect to
assessing impact, can be gained through full content analysis. The key
challenge, however, is that this analysis is currently a manual effort such that it can not be performed for all publications, but rather only for a select few for a specific purpose.
In the work of [9][7],
a new approach called semantometrics was established as a first step
toward leveraging automation of full content analysis for impact
assessment. In [9],
semantometrics is used to automatically quantify how well a
cited/citing pair "creates a "bridge" between what we already know and
something new". If the similarity of the content between the pair is
high, then the "bridge" is likely to be very short. If the similarity is
low, then the "bridge" is likely to be very long. Longer bridges then
are considered to have high impact values. While not citing the
semantometric approach first developed in [9], the work of [20] confirmed the effectiveness of this approach. In the work of [7],
semantometrics is used to automatically quantify the diversity of
collaboration between co-authors referred to as research endogamy.
Higher values of endogamy imply low cross-disciplinary efforts, and vice
versa. Further, using semantometrics to investigate co-authorship and
endogamy provided evidence to support that "collaboration across
disciplines happens more often on a short-term basis".
We posit that this prior work should be further extended in order to
more fully assess scientific impact at a higher resolution of detail
than what is currently capable with citation analysis. In our assessment
of publications that have acknowledged using the Oak Ridge Leadership
Computing Facility (OLCF), we have identified at least two areas of
opportunity to leverage full content analysis: 1) context-aware citation
analysis and 2) leading edge impact assessment.
4.1 Context-Aware Citation Analysis
Depending on the context of the work performed, not all citations convey equal impact to a citing work.As a specific example, the work of [21] cites the work of [8], which in turn, acknowledges having used OLCF resources. [21] cites [8] in the following way, "We used the Community Earth System Model28 to simulate UHI." From this context and the scope of the work performed in [21], it can be concluded that the work of [21] most likely could not have been performed if not for the work performed by [8]. Further, a possible conclusion could also be drawn that the work of [8]
could not have been performed if not for the availability and existence
of a resource such as OLCF, although the evidence for this is not as
strong as it's not clear whether that work could have been performed
with another resource of the same type.
In contrast, the work of [21] also cites the work of [6]
in the following way, "A measure of heatwave intensity is the degree of
deviation, in multiples of standard deviation (North American mean
value, s < 0.6 K) of summertime temperature from the climatological
mean21." From this context and the scope of work performed in [21], it can be concluded that this citation is simply used as reference material for the reader. If the work of [6] had not been cited, the value of the work performed in [21] would likely not be diminished.
Unfortunately, there does not appear to be a current, automated
method to address this discrepancy in the way publications are cited.
Only through the context of the full content does the discrepancy
appear, and traditional means of citation analysis could possibly hide
the value of a higher impact of one cited work over another.
4.2 Leading Edge Impact Assessment
Scientific research areas often "ebb and flow" as progress is made.Unfortunately, as a result of the noise from an increasing number of
scientific publications annually, the probability of appropriately
citing relevant works diminishes each year. This leads to a severely
restricted ability to observe the "ebb and flow"of research areas
through citation analysis alone. Consequently, assessment of impact is
also restricted. We postulate that the ebb and flow of research areas
likely resembles the Everett Rogers' Innovation Adoption Lifecycle [18]
as shown in Figure 1. Seminal papers would fall within the leftmost
leading edge of innovators, and based on interest in the research area,
the number of papers resulting from the seminal paper would grow until
the research area no longer remains of interest or morphs into another
topic.
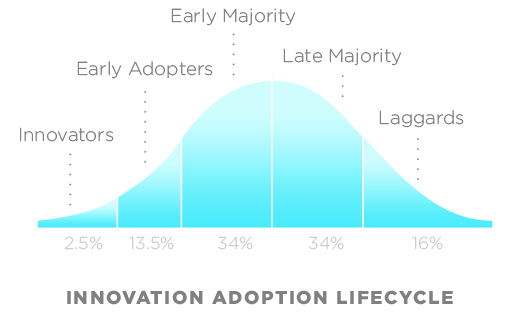
Figure 1: Everett Rogers' Innovation Adoption Lifecycle model [18]
(Licensing information for this figure is available from Wikipedia here.)
of inadequate citations of appropriate publications, a publication could
be assessed based on its relative position within this lifecycle model.
The closer to the leftmost leading edge of the model, the higher the
impact in that the publication has the opportunity to shape the field.
To evaluate this conjecture, we randomly selected a publication from
2009 [15]
that acknowledged having used resources of the Oak Ridge Leadership
Computing Facility (OLCF). This publication, entitled "Systematic
assessment of terrestrial biogeochemistry in coupled climate—carbon
models", is focused on research involving the carbon cycle, and was
published in the journal Global Change Biology. Abstracts from every article published in this journal from 2009 to
2015 were collected. These abstracts were then clustered using techniques described in [17][16].
For 2009, we cluster all of the abstracts and observed that a cluster
formed of abstracts that are topically oriented around the "carbon
cycle". This cluster contains the [15] paper. For each year after 2009, we cluster all the abstracts for the respective year along with the [15] paper. The [15]
paper continues to cluster within the "carbon cycle" group for each
year, and we count the number of publications within that cluster for
each year. The plot for this is shown in Figure 2.
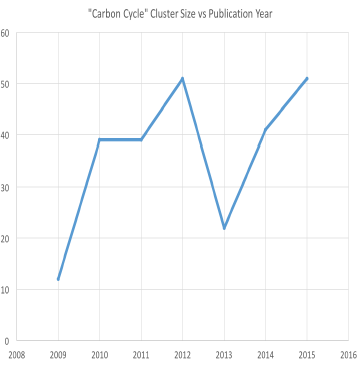
Figure 2: Cluster Size vs Publication Year for publications relating to the "Carbon Cycle"
a severe decline and then resurgence of the cluster size. While not an
entirely conclusive result, the growth and decline does seem to emulate
the lifecycle model of Figure 1. Future work will examine additional
journals beyond Global Change Biology to determine if this growth
and decline is evident in other journals, thereby representative of the
research field, or if not evident, simply a reflection of submissions
and acceptance rate of Global Change Biology. Based on this
initial result, an impact assessment could incorporate the location in
time of when paper was published with respect to the
growth and decline of the topic cluster. In this example, the [15]
paper occurred at the leftmost leading edge of the curve, thus
increasing its ability to impact the field even though it may not
receive appropriate citing and defining it as a more innovating paper
relative to the rest of the field.
5 Summary
This work highlights some of the issues involving impact assessmentof publications when using citation analysis and the need to more
clearly define "impact". In addition, we emphasize the need for better
metrics that leverage full content analysis of publications. Analysis of
the full content reveals additional detail of how citations differ
where some papers are cited simply as references while others are
absolutely critical to the citing work, and that the topics with respect
to when the paper is published provide additional insight into possible
impact. We posit that further work should be performed to leverage full
content analysis in two ways. First, a context-aware citation analysis
that takes into account that the citations are have different value to a
citing work and should be a factor in assessing impact. Second, a
leading edge impact assessment from the full content can reveal how
quickly a research area begins, grows, and fades, and that the timing of
the publication should be a factor in assessing impact. Future work
will expand on these areas in support of more clearly evaluating
publication impact on science and technology.
Acknowledgements
This manuscript has been authored by UT-Battelle, LLC and usedresources of the Oak Ridge Leadership Computing Facility at the Oak
Ridge National Laboratory under Contract No. DE-AC05-00OR22725 with the
U.S. Department of Energy. The United States Government retains and the
publisher, by accepting the article for publication, acknowledges that
the United States Government retains a non-exclusive, paid-up,
irrevocable, world-wide license to publish or reproduce the published
form of this manuscript, or allow others to do so, for United States
Government purposes. The Department of Energy will provide public access
to these results of federally sponsored research in accordance with the
DOE Public Access Plan.
References
[1] | ARK (archival resource key) identifiers, 2015. |
[2] | Aesis: Network for advancing & evaluating the societal impact of science, 2016. |
[3] | Snowball Metrics: Global standards for institutional benchmarking, 2016. |
[4] | N. A. Ebrahim, H. Salehi, M. A. Embi, F. Habibi, H. Gholizadeh, S. M. Motahar, and A. Ordi. Effective strategies for increasing citation frequency. International Education Studies, 6(11):93-99, October 2013. http://doi.org/10.5539/ies.v6n11p93 |
[5] | B. Godin and C. Dore. Measuring the impacts of science; beyond the economic dimension, 2005. http://doi.org/10.1038/embor.2012.99 |
[6] | J. Hansen, M. Sato, and R. Ruedy. Perception of climate change. Proceedings of the National Academy of Sciences USA, 109(37):E2415-23, Sep 2012. http://doi.org/10.1073/pnas.1205276109 |
[7] | D. Herrmannova and P. Knoth. Semantometrics in coauthorship networks: Fulltext-based approach for analysing patterns of research collaboration. D-Lib Magazine, 21(11). http://doi.org/10.1045/november2015-herrmannova |
[8] | J. W. Hurrell, M. M. Holland, P. R. Gent, et al. The community earth system model: A framework for collaborative research. Bulletin of the American Meteorological Society, 94(9), 2013. http://doi.org/10.1175/BAMS-D-12-00121.1 |
[9] | P. Knoth and D. Herrmannova. Towards semantometrics: A new semantic similarity based measure for assessing a research publication's contribution. D-Lib Magazine, 20(11). http://doi.org/10.1045/november2014-knoth |
[10] | P. O. Larsen and M. von Ins. The rate of growth in scientific publication and the decline in coverage provided by science citation index. Scientometrics, 84(3):575-603, September 2010. http://doi.org/10.1007/s11192-010-0202-z |
[11] | M. H. MacRoberts and B. R. MacRoberts. Problems of citation analysis. Scientometrics, 36(3):435-444. http://10.1007/BF02129604 |
[12] | M. H. MacRoberts and B. R. MacRoberts. Problems of citation analysis: A critical review. Journal of the American Society for Information Science, 40(5):342-349, 1989. http://doi.org/10.1002/(SICI)1097-4571(198909)40:5<342::AID-ASI7>3.0.CO;2-U |
[13] | L. I. Meho. The rise and rise of citation analysis. Physics World, 20(1), January 2007. http://doi.org/10.1088/2058-7058/20/1/33 |
[14] | J. Priem, D. Taraborelli, P. Groth, and C. Neylon. Altmetrics: A manifesto, 2010. |
[15] | J. T. Randerson, F. M. Hoffman, P. E. Thorton, N. M. Mahowald, K. Lindsay, Y.-h. Lee, C. D. Nevison, S. C. Doney, G. Bonan, R. Stockli, C. Covey, S. W. Running, and I. Y. Fung. Systematic assessment of terrestrial biogeochemistry in coupled climate—carbon models. Global Change Biology, 15(10):2462-2484, 2009. http://doi.org/10.1111/j.1365-2486.2009.01912.x |
[16] | J. W. Reed, Y. Jiao, T. E. Potok, B. Klump, M. Elmore, and A. R. Hurson. Tf-icf: A new term weighting scheme for clustering dynamic data streams. In Proceedings of 5th International Conference on Machine Learning and Applications (ICMLA'06), pages 1258-1263. IEEE, 2006. |
[17] | J. W. Reed, T. E. Potok, and R. M. Patton. A multi-agent system for distributed cluster analysis. In Proceedings of Third International Workshop on Software Engineering for Large-Scale Multi-Agent Systems (SELMAS'04) W16L Workshop — 26th International Conference on Software Engineering, pages 152-155. IEEE, 2004. |
[18] | E. Rogers. Diffusion of Innovations, 5th Edition. Simon and Schuster, 2003. |
[19] | W. J. Sutherland, D. Goulson, S. G. Potts, and L. V. Dicks. Quantifying the impact and relevance of scientific research. PLoS ONE, 6(11), 2011. http://doi.org/10.1371/journal.pone.0027537 |
[20] | R. Whalen, Y. Huang, C. Tanis, A. Sawant, B. Uzzi, and N. Contractor. Citation distance: Measuring changes in scientific search strategies. In Proceedings of the 25th International Conference Companion on World Wide Web, WWW '16 Companion, pages 419-423, Republic and Canton of Geneva, Switzerland, 2016. International World Wide Web Conferences Steering Committee. |
[21] | L. Zhao, X. Lee, R. B. Smith, and K. Oleson. Strong contributions of local background climate to urban heat islands. Nature, 511(7508):216-219, 07 2014. http://doi.org/10.1038/nature13462 |
About the Authors
Robert M. Patton received his PhD in Computer
Engineering with emphasis on Software Engineering from the University of
Central Florida in 2002. He joined the Computational Data Analytics
group at Oak Ridge National Laboratory (ORNL) in 2003. His research at
ORNL has focused on nature-inspired analytic techniques to enable
knowledge discovery from large and complex data sets, and has resulted
in approximately 30 publications pertaining to nature-inspired analytics
and 3 patent applications. He has developed several software tools for
the purposes of data mining, text analyses, temporal analyses, and data
fusion, and has developed a genetic algorithm to implement maximum
variation sampling approach that identifies unique characteristics
within large data sets.
Engineering with emphasis on Software Engineering from the University of
Central Florida in 2002. He joined the Computational Data Analytics
group at Oak Ridge National Laboratory (ORNL) in 2003. His research at
ORNL has focused on nature-inspired analytic techniques to enable
knowledge discovery from large and complex data sets, and has resulted
in approximately 30 publications pertaining to nature-inspired analytics
and 3 patent applications. He has developed several software tools for
the purposes of data mining, text analyses, temporal analyses, and data
fusion, and has developed a genetic algorithm to implement maximum
variation sampling approach that identifies unique characteristics
within large data sets.
Christopher G. Stahl recently graduated with a
Bachelor of Science from Florida Southern College. For the past year he
has been participating in the Higher Education Research Experiences
(HERE) program at Oak Ridge National Laboratory. His major research
focuses on data mining, and data analytics. In the future he plans on
pursing a PhD in Computer Science with a focus on Software Engineering.
Bachelor of Science from Florida Southern College. For the past year he
has been participating in the Higher Education Research Experiences
(HERE) program at Oak Ridge National Laboratory. His major research
focuses on data mining, and data analytics. In the future he plans on
pursing a PhD in Computer Science with a focus on Software Engineering.
Jack C. Wells is the director of science for the
National Center for Computational Sciences (NCCS) at Oak Ridge National
Laboratory (ORNL). He is responsible for devising a strategy to ensure
cost-effective, state-of-the-art scientific computing at the NCCS, which
houses the Department of Energy's Oak Ridge Leadership Computing
Facility (OLCF). In ORNL's Computing and Computational Sciences
Directorate, Wells has worked as group leader of both the Computational
Materials Sciences group in the Computer Science and Mathematics
Division and the Nanomaterials Theory Institute in the Center for
Nanophase Materials Sciences. During a sabbatical, he served as a
legislative fellow for Senator Lamar Alexander, providing information
about high-performance computing, energy technology, and science,
technology, engineering, and mathematics education issues.Wells began
his ORNL career in 1990 for resident research on his Ph.D. in Physics
from Vanderbilt University. Following a three-year postdoctoral
fellowship at Harvard University, he returned to ORNL as a staff
scientist in 1997 as a Wigner postdoctoral fellow. Jack is an
accomplished practitioner of computational physics and has been
supported by the Department of Energy's Office of Basic Energy Sciences.
Jack has authored or co-authored over 70 scientific papers and edited 1
book, spanning nanoscience, materials science and engineering, nuclear
and atomic physics computational science, and applied mathematics.
National Center for Computational Sciences (NCCS) at Oak Ridge National
Laboratory (ORNL). He is responsible for devising a strategy to ensure
cost-effective, state-of-the-art scientific computing at the NCCS, which
houses the Department of Energy's Oak Ridge Leadership Computing
Facility (OLCF). In ORNL's Computing and Computational Sciences
Directorate, Wells has worked as group leader of both the Computational
Materials Sciences group in the Computer Science and Mathematics
Division and the Nanomaterials Theory Institute in the Center for
Nanophase Materials Sciences. During a sabbatical, he served as a
legislative fellow for Senator Lamar Alexander, providing information
about high-performance computing, energy technology, and science,
technology, engineering, and mathematics education issues.Wells began
his ORNL career in 1990 for resident research on his Ph.D. in Physics
from Vanderbilt University. Following a three-year postdoctoral
fellowship at Harvard University, he returned to ORNL as a staff
scientist in 1997 as a Wigner postdoctoral fellow. Jack is an
accomplished practitioner of computational physics and has been
supported by the Department of Energy's Office of Basic Energy Sciences.
Jack has authored or co-authored over 70 scientific papers and edited 1
book, spanning nanoscience, materials science and engineering, nuclear
and atomic physics computational science, and applied mathematics.
Measuring Scientific Impact Beyond Citation Counts
No comments:
Post a Comment